Harnessing Generative AI securely – from creation to caution
Gaining a competitive advantage is a compelling motivator for companies to adopt AI. But security remains a concern
Written by Keith Batterham
Not a day goes by without learning of new use cases for artificial intelligence and machine learning. It feels like a firehose of information and opinions that are equally inspiring and terrifying. But no matter which type of opinion you align with, the technological genie is well and truly out of the bottle.
Whether you’re a multi-national organisation, a start-up, an established company looking for growth and efficiency, or even someone wondering what all this is about, if you’re not already using or planning to use AI technologies, it’s likely that your peers are.
Gaining a competitive advantage is a compelling motivator and yet, especially with businesses, you must consider security issues such as a potential lack of transparency and explainability of results, meaning they may be difficult to audit and trust. Of course, there are also well documented examples of exploiting the way in which these systems work, using techniques to alter behaviour and bypass safeguards.
A quick definition
Generative AI tools are algorithms that can create new content, such as text, images, audio, video, code, and simulations based on input data. They are powered by machine learning models that are generally trained on large amounts of data to learn patterns and generate outputs that mimic the original data, giving the potential to revolutionise industries and domains, from entertainment to education to healthcare.
These tools can help create new and engaging content, enhance existing content, optimise business processes, and solve complex problems. The quality, accuracy, and speed of responses will improve dramatically over time.
Probably the most impactful example of generative AI that I’ve seen implemented by clients so far has been within data analytics and risk mitigation. Here they have used synthetic data to explore and understand complex datasets, and then harnessed this understanding to analyse patterns and behaviours of transactions or users. Increasingly I’m seeing experiments into code generation and the documentation of legacy code to aid in maintenance or refactoring.
Be aware
It can be really tempting to jump right into generative AI to gain those competitive advantages that these powerful technologies can offer, but be aware of the challenges and risks to enable you to do this safely and responsibly. From a security and risk perspective, at a bare minimum consider:
- Technical complexity and debt – With potentially billions or trillions of parameters, these can be difficult to understand, debug, and optimise with significant compute, storage, and infrastructure needs. Equally they may not be compatible with existing systems or processes so may require integration, adaptation, or transformation. Like with introducing any other new technology, new dependencies, vulnerabilities, and security threats will need to be managed.
- Monitoring for potential misuse – Malicious actors may be able to generate harmful or misleading content, such as fraud, cyberattacks, or misinformation. They may also produce inaccurate or inappropriate content due to errors, biases, or limitations in their data or algorithms. This can compromise the integrity or authenticity of these systems or their outputs, leading to security breaches or losses.
- Legal concerns and algorithmic bias – Concerns such as data privacy, copyright, or intellectual property rights are inevitable since biased or discriminatory content may be generated that affects the fairness, accountability, or transparency of their outcomes. These can undermine the trust or confidence of systems or their users, leading to legal disputes or reputational risks.
Don’t have in-house expertise on AI and security? We can help
Be proactive
Businesses should adopt a holistic and proactive approach to generative AI security risk management. This includes:
- Understanding the basics of how these tools work, what they can do, and what they cannot do and selecting those that suit your desired outcomes and success criteria based on your budget and capabilities.
- Educating and training staff and stakeholders on the benefits and risks of these new technologies, and implementing best practices and standards for development, deployment, and governance.
- Experimenting on a small scale before scaling up, taking care to evaluate the quality and relevance of the generated outputs, and checking for any errors or issues. Use tools and techniques to test, monitor, and improve security posture.
- Respecting the data rights and privacy of others; verifying and attributing the content you use or produce, collaborating with human experts or peers, and following legal and ethical guidelines.
Be prepared
If you’re in an organisation, now is the time to revisit your threat modelling, technology use and access policies and risk documentation, as it’s the perfect opportunity to bring together the business, technology and security. Many of the products and services you currently use either already have or will have AI and ML elements embedded, so you need to determine how you want to embrace or restrict them.
A final thought
As a security professional, my work involves being able to understand how strategies are supported by technologies, and how to implement them safely without introducing unnecessary friction. These are exciting and powerful technologies that can help both individuals and organisations create new value and opportunities, but they also require careful and responsible use to avoid potential pitfalls and risks. By following the steps laid out, you can start your journey and embrace the new generative AI tools safely and responsibly.
Get in touch today to see how we can help you manage your AI security risks
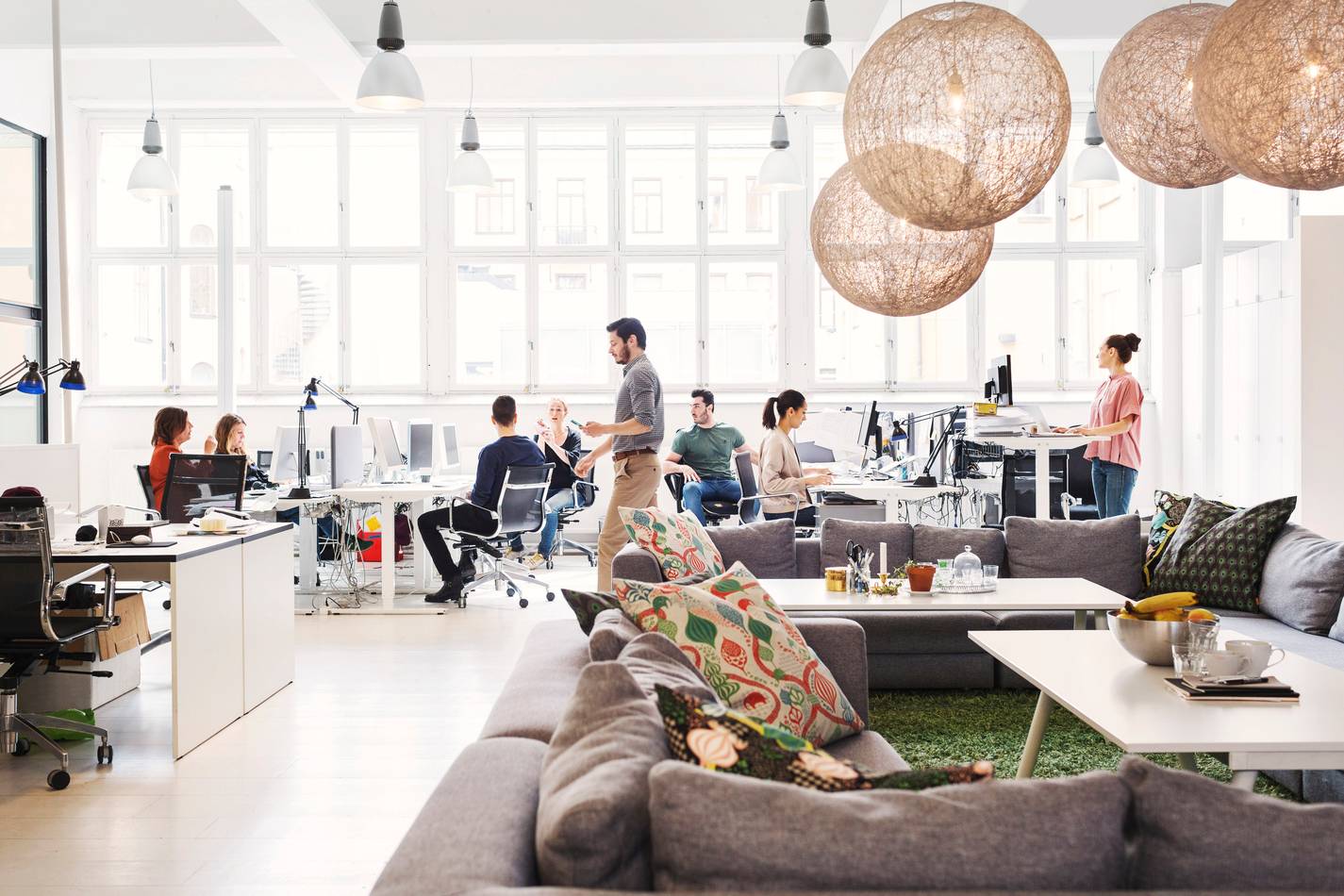
Question?
Our specialists have the answer